DIY IIoT Revolution in Smart Factories
- Sanjeev Datla
- Jan 3, 2024
- 9 min read
How a new generation of user-friendly, low-code IoT data management technologies is transforming engineers of any skill level into "citizen data scientists".
Overview
The concept of 'smart factories' has quickly evolved from a futuristic vision to a realistic reality in the arena of modern manufacturing. These advanced manufacturing environments, defined by their use of cutting-edge technologies, are altering the very fabric of manufacturing industry.
The Internet of Things (IoT) is at the center of this change, a network of interconnected devices and sensors that communicate with one another to make real-time, data-driven decisions. The Internet of Things (IoT) lies at the heart of smart factories, orchestrating a symphony of machines, analytics, and human skills to produce a more efficient, productive, and intelligent manufacturing process.
However, incorporating IoT into the production landscape is fraught with difficulties. Traditionally, companies relied on outsourcing development of custom IoT solutions or investing in expensive solutions from legacy leaders, which frequently resulted in significant recurring costs and vendor lock-in problems. While effective, these approaches can be rigid and may fail to meet the specific, ever-changing needs of a dynamic manufacturing environment.
The era of user-friendly, ready-to-use IoT data management tools is a major game changer in the world of smart manufacturing. These tools signify a movement toward empowering engineers to create cost-effective and adaptive custom-tailored solutions. By leveraging these readily available technologies, engineers and manufacturers can break free from the limits of traditional approaches, avoiding the frustrations of costly subscriptions and the limitations imposed by closed, siloed ecosystems.
This article dives into how IoT and IoT data management acts as the foundational layer in smart factories. It explores how user-friendly, low-code IoT data management tools are enabling engineers to develop and adapt like never before. We will examine the advantages of a do-it-yourself (DIY) approach to data collecting, data integration, and analytics, as well as the limits of standard compartmentalized technologies. Our journey will demonstrate how these adaptable tools enable any engineer, whether in production, control systems, or maintenance, to become data-driven, opening the road for smarter, more efficient factories.
The Internet of Things Foundation in Smart Factories
Understanding the Role of IoT
In the context of modern manufacturing, the Internet of Things (IoT) is more than just a term; it is the foundation upon which smart factories are created. At its heart, IoT in smart factories is a network of sensors and equipment that are interconnected and communicate with one another. This network gathers massive amounts of data from diverse factory operations, which is then utilized to optimize procedures, decrease downtime, and boost efficiency.

The diagram shows the importance of IoT in smart factories. It represents IoT and Connectivity as the base layer, indicating its fundamental role. Various technologies are placed below this layer, including Wireless Sensor Networks, Data Collection & Management, and Big Data Analytics, all of which rely on the data and connectivity supplied by IoT. This hierarchy shows how IoT supports every sophisticated technology used in a smart factory, from robotics and AI and machine learning to cybersecurity and energy management.
Use Cases
![]() | ![]() | ![]() |
Automotive Industry Transformation: IoT sensors can be placed across the manufacturing floor of a large automotive factory to monitor equipment performance. This real-time data is utilized to predict machine faults before they occur, lowering unexpected downtime and maintenance costs dramatically. Furthermore, IoT-enabled robotics can optimize assembly lines, increasing speed and precision. | Energy Efficiency in Textile Manufacturing: An IoT solution installed by a textile producer can optimize energy consumption. Sensors monitor real-time energy consumption, and data analytics are utilized to discover patterns and locations of high energy consumption. The plant can achieve significant reductions in energy costs and carbon footprint by modifying processes and machinery based on these observations. | Pharmaceutical Industry Compliance and Quality Control: Strict compliance and quality control are critical in the pharmaceutical industry. An IoT-powered smart factory here can use sensors to continuously monitor environmental factors such as temperature and humidity, which is critical for product integrity. The information gathered guarantees that health rules are followed and that product quality is constant. |
These use cases demonstrate IoT's transformational power across manufacturing sectors. IoT acts as the foundational layer, improving operational efficiency and opening up new opportunities for innovation, sustainability, and compliance adherence.
The Emergence of User-Friendly, Low-Code IoT Data Management Tools
Difficulties with Traditional Approaches
Traditional techniques to implement IoT solutions in manufacturing frequently include outsourcing crucial development or depending on proprietary tools from veteran corporations. While these methods have their advantages, they are not without restrictions and costs:
High Expense: Tools from legacy leaders are frequently expensive, requiring a significant financial investment. In contrast, outsourcing incurs recurring expenditures that might add up over time.
Vendor Lock-In: Relying on a legacy, proprietary tools can result in a dependency that limits flexibility. Factories may become locked in a loop of exclusively employing specific products, limiting their ability to adapt and expand with evolving technologies.
Lack of Customization: Typically, legacy data tools are limited to the vendors platforms and generic. They may fail to meet the specific needs of a certain factory. Feature requests may take months or even years, resulting in inefficiencies and a less-than-optimal fit for specific operational requirements.
Benefits of DIY IoT Data Management Tools
In contrast, the availability of user-friendly, ready-to-use IoT data tools has been a major changer, providing various benefits:
Adaptability: These tools are intended to be configurable and adaptable, allowing factories to tailor them to their individual requirements. This adaptability allows for a more customized approach to problem solutions and process optimization.
Cost-Effectiveness: Because they are less expensive, ready-to-use IoT data management tools enable smart factory technologies available to a broader spectrum of manufacturers, including small and medium-sized businesses.
Independence from Vendor Lock-Ins: Turnkey IoT data management tools leverage open standards and interfaces to maximize interoperability, manufacturers avoid being tied to a single vendor, allowing them to select the best solutions at any given time and to switch as newer, more efficient technologies emerge.
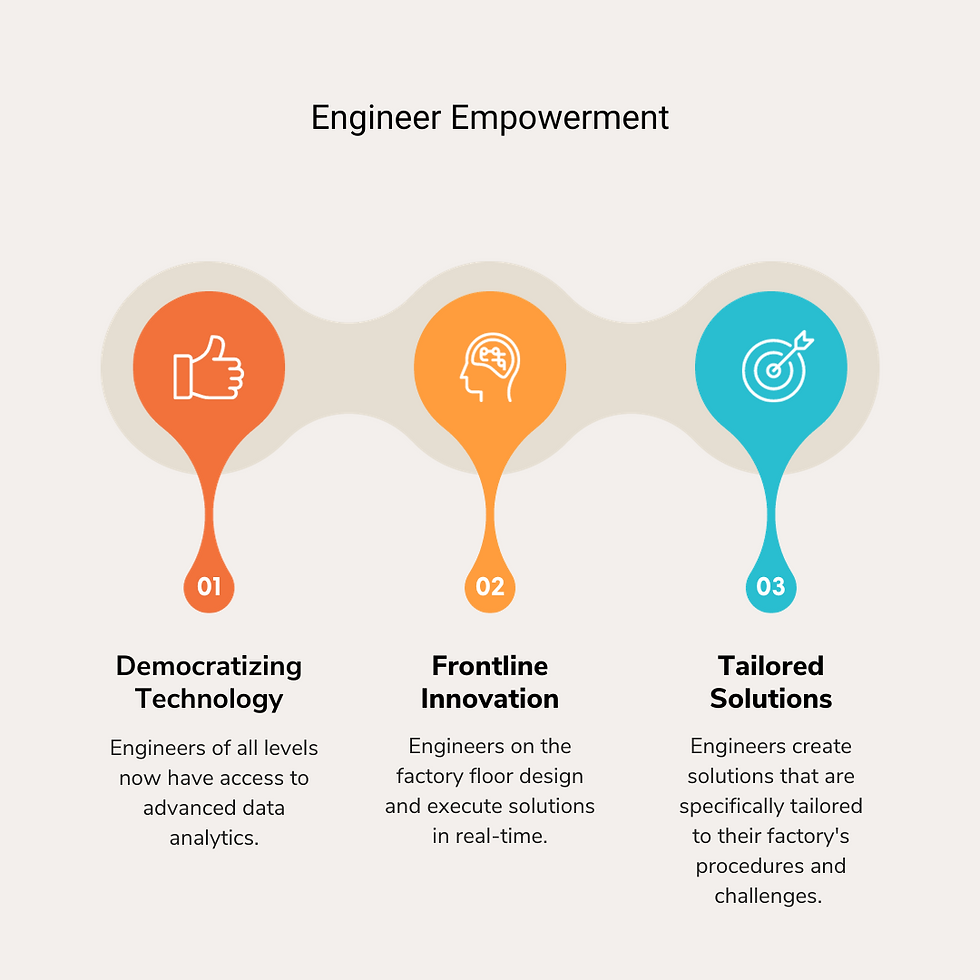
The rise of user-friendly, low-code IoT data management tools signifies a fundamental shift in the smart manufacturing technology environment. These technologies are unleashing new potentials in manufacturing efficiency, creativity, and adaptation by giving an alternative to existing, often limited approaches.
Do-It-Yourself Data Collection and Integration
Personalized Data Solutions
Do-It-Yourself (DIY) data gathering and integration in smart factories represents a big step toward autonomy and customization. Engineers can now design bespoke data solutions tailored to their specific operational requirements. This capability is critical in a number of ways:
Targeted Data collecting: Instead of depending on generic, one-size-fits-all solutions, engineers may create data collecting systems that focus on the most relevant data points for their individual operations.
Seamless Integration: DIY tools allow data collecting to be seamlessly integrated with current systems, allowing a smooth flow of information across multiple platforms and departments within the plant.
Real-Time Adjustments: The ability to change and adapt data gathering parameters in real time enables more responsive and dynamic data management, resulting in more agile production processes.
Breaking Down Barriers
Traditional data management solutions frequently result in the data silos, or segmented and isolated information. DIY data collecting and integration approaches provide a solution:
Unified Data Streams: Engineers may ensure that data from diverse sources and systems is consolidated, resulting in a unified view of the factory's operations by developing custom solutions.
Improved Collaboration: A uniform data presentation promotes collaboration across many teams by making data easily available and usable across departments.
Holistic Decision-Making: Breaking down silos leads to a more holistic approach to decision-making, where data from all elements of the production is taken into account, resulting in more informed and effective strategies.
Advantages of Do-It-Yourself Data Management
The do-it-yourself approach to data management in smart factories has several significant advantages:
Cost Savings: DIY solutions frequently need a cheaper investment than established tools from legacy leaders, especially when long-term costs connected with subscriptions and licensing fees are included.
Increased Flexibility: Engineers may easily adapt and alter their data management systems to meet changing needs and conditions, as opposed to being constrained by vendor-specific limits or purchasing additional, expensive licenses.
Enhanced Data Control: DIY data management returns control to the factory, allowing for greater customisation in how data is collected, stored, and used. This level of control is critical in a world where data is increasingly regarded as a valuable asset.
Scalability: DIY solutions may expand and adapt alongside the production, ensuring that the data management system stays relevant and successful as the firm grows or shifts its focus.
In essence, the DIY data collecting and integration approach enables smart factories to be more responsive, efficient, and data-driven. It signifies a shift away from reliance on external suppliers and toward self-sufficiency, in which manufacturers have the skills and competencies to manage their data ecosystem in a way that best matches their particular operational landscapes.
DIY Analytics vs. Vendor-Specific Analytics in Smart Factories
Comparative Evaluation
The change from traditional analytics tools from industry veterans to nimble DIY analytics in smart factories represents a substantial shift in data processing and utilization. This shift has significant ramifications for cost, flexibility, and effectiveness:
Flexibility and modification: Because traditional tools offer limited modification, companies must adjust their processes to the tool's capabilities. DIY analytics, on the other hand, are designed to be adaptable, allowing factories to adjust the analytics to their own needs and processes.
Effectiveness and Efficiency: While standard tools are generally trustworthy and robust, they may not always provide the precise insights required for the operations of a specific manufacturing. DIY analytics tools can be tailored to address unique operational issues, potentially providing more relevant and actionable insights.
Use Cases
Several real-world examples demonstrate the utility of DIY analytics in smart factories:
Optimized Production Line: A mid-sized automobile components firm can optimize their production line using DIY analytics. The firm can detect bottlenecks and inefficiencies by designing a unique analytics solution that combines data from multiple phases of the manufacturing process, resulting in a 20% boost in production efficiency.
Reduced Energy Consumption: A textile plant can utilize a DIY analytics tool to monitor and evaluate energy consumption across its activities. The customized tool can assist in identifying patterns of excessive energy use, which result in targeted actions that can decrease energy expenses by 15% while preserving industrial output.
Improved Quality Control: To strengthen its quality control systems, a consumer electronics manufacturer can deploy DIY analytics. The company can discover and address errors more rapidly by evaluating data from the assembly line in real time, resulting in a 30% reduction in product returns due to manufacturing defects.
These examples show the real-world benefits of implementing DIY analytics in smart manufacturing. These demonstrate the opportunity to boost efficiency, cut costs, and improve product quality by adopting unique solutions suited to their specific needs, demonstrating the potential of DIY analytics as a powerful tool in the modern manufacturing scene.
Ready-to-Use, Customizable IoT Data Management Tools in a Variety of Engineering Fields
Cross-Disciplinary Versatility
DIY IoT data management tools have altered the smart factory scene by providing diverse solutions that go beyond traditional manufacturing. Their adaptability and utility in tackling a wide range of difficulties is demonstrated by their applicability across numerous engineering disciplines:
Manufacturing: These tools provide real-time monitoring of production lines in the manufacturing sector, allowing for fast adjustments to boost efficiency and eliminate waste.
Control Systems: These tools are used by engineers to create more responsive and adaptive control systems, resulting in improved automation and process control.
Maintenance: These tools' predictive maintenance capabilities aid in recognizing probable equipment faults before they occur, reducing downtime and increasing the life of machinery.
Quality Assurance: Ready-to-use technologies help maintain high-quality standards by combining data from numerous checkpoints, allowing for speedier detection and rectification of defects.
Ready-to-use, customizable IoT data management tools enable engineers to develop and optimize processes in their respective areas by delivering flexible, cost-effective, and efficient solutions, paving the way for more intelligent and responsive smart factories.
The Advantages of a Data-Driven Engineering Approach
Improved Decision-Making
The shift to a data-driven approach considerably improves decision-making capabilities in smart factories. This transition entails relying on massive amounts of data to make informed, strategic decisions:
Accurate Predictions and Planning: Factory managers can use data-driven models to forecast market trends, production needs, and potential bottlenecks, allowing for proactive planning and resource allocation.
Real-Time Problem Solving: Engineers can immediately detect and address issues as they arise with real-time data at their fingertips, decreasing downtime and enhancing overall productivity.
Informed Strategic Decisions: Long-term strategic decisions, like as investments in new technologies or capacity expansions, are made more dependable and anchored in concrete data analysis, lowering risk and increasing success potential.
Operations Simplified
The integration of data collecting and analytics tools improves manufacturing operations in a number of critical areas:
Optimizing Production Flows: Through the analysis of production data, companies can uncover inefficiencies in their workflows and create more streamlined processes.
Resource Optimization: Data-driven approaches enable more efficient use of resources such as materials and energy, resulting in cost savings and increased sustainability.
Supply Chain Management: Improved data on supply and demand dynamics aids in the optimization of inventory levels and logistics, ensuring materials are available when needed, and eliminating waste.
Promoting Innovation
A data-driven engineering strategy is an effective driver of innovation in smart factories:
New Product Development: Data analytics insights can be used to inform the development of new goods that better satisfy market needs and client preferences.
Process Innovation: Continuous production data analysis can lead to revolutionary manufacturing methods, potentially disrupting existing procedures.
Customization and Personalization: Data-driven insights enable higher customization and personalization in manufacturing, providing a competitive advantage in markets where these elements are becoming increasingly important to customers.
Finally, in smart factories, a data-driven engineering approach leads to better informed decision-making, simpler operations, and a fertile environment for innovation. Smart factories can attain higher levels of efficiency, agility, and competitiveness in the continuously changing industrial landscape by harnessing the power of data.
Future IoT Trends and Forecasts in Smart Factories

Changing Technology Environment
The landscape of IoT and smart factory technologies is constantly evolving, driven by rapid technological breakthroughs and changing industry needs. Several significant advancements can be expected in the future:
Increased IoT Integration: In the future, IoT devices will likely be integrated even more deeply into manufacturing processes, with more advanced sensors and linked devices becoming typical in factories.
AI and ML Advancements: AI and machine learning will continue to progress, allowing for more nuanced and complex data analysis. This will result in even better predictions and automated decision-making.
Emergence of 5G Technology: The deployment of 5G networks will dramatically improve IoT capabilities in smart factories by allowing for quicker and more reliable connections, allowing for real-time data transfer and processing.
DIY IoT Data Management Tools' Expanding Role
The role of user-friendly, low-code, customizable IoT data management tools in the smart manufacturing landscape is expected to grow significantly:
Citizen Data Scientists: There will be an increase in 'citizen data scientists' - professionals who, while not formally trained in data science, use these DIY data management tools to undertake major data analysis and contribute to decision-making processes.
Widespread Adoption Across Industries: As these tools grow more user-friendly and adaptable, we may expect their use to wide beyond traditional manufacturing.
Collaborative Ecosystems: In the future, more collaborative ecosystems may emerge, where products from different manufacturers can interact seamlessly, increasing flexibility and innovation.
Conclusion
IoT is the linchpin, fueling a slew of technological improvements. The proliferation of ready-to-use and DIY IoT data management tools has democratized access to advanced data management and analytics capabilities, empowering engineers and manufacturers at all levels.
These tools represent a paradigm shift in how manufacturers function and innovate. Manufacturers can achieve new levels of efficiency, adaptability, and inventiveness by embracing these solutions.
The future of manufacturing is data-driven, networked, and innovative, and factories can position themselves at the vanguard of this exciting new era by embracing the power of IoT and ready-to-use, customizable IoT data management solutions.
Test drive Machinechat's user-friendly, low code IoT data management tools built for engineers of any skill level.
Comments